Nov 5, 2024
Aditya
Gaur
Picture this: a marketing associate at a large enterprise finds herself juggling repetitive tasks—email drafting, social media posts, content planning. In her search for efficiency, she starts using an AI tool to automate some of these tasks. Meanwhile, an engineer in another department leverages ChatGPT to assist with code generation. Within weeks, these AI tools, sourced outside of official channels and beyond the IT department’s oversight, are being adopted across the organization by employees eager to streamline their workloads.
This growing use of unauthorized AI applications in the workplace—known as Shadow AI—parallels the rise of Shadow IT but introduces even more significant risks. Shadow AI refers specifically to generative and machine learning applications used without IT’s knowledge or approval. With AI tools more accessible than ever and their capabilities expanding, Shadow AI poses a significant, rapidly scaling cybersecurity threat.
Why Shadow AI Has Emerged So Quickly
Fueled by the ease of access to free or low-cost generative AI tools available right in any browser tab, Shadow AI is emerging as a silent disruptor across industries. According to Salesforce, 49% of employees have used generative AI, with over half saying their usage is increasing since they first adopted the technology. Employees leverage AI for everything from drafting emails and summarizing reports to creating data visualizations, often unaware of the security implications.
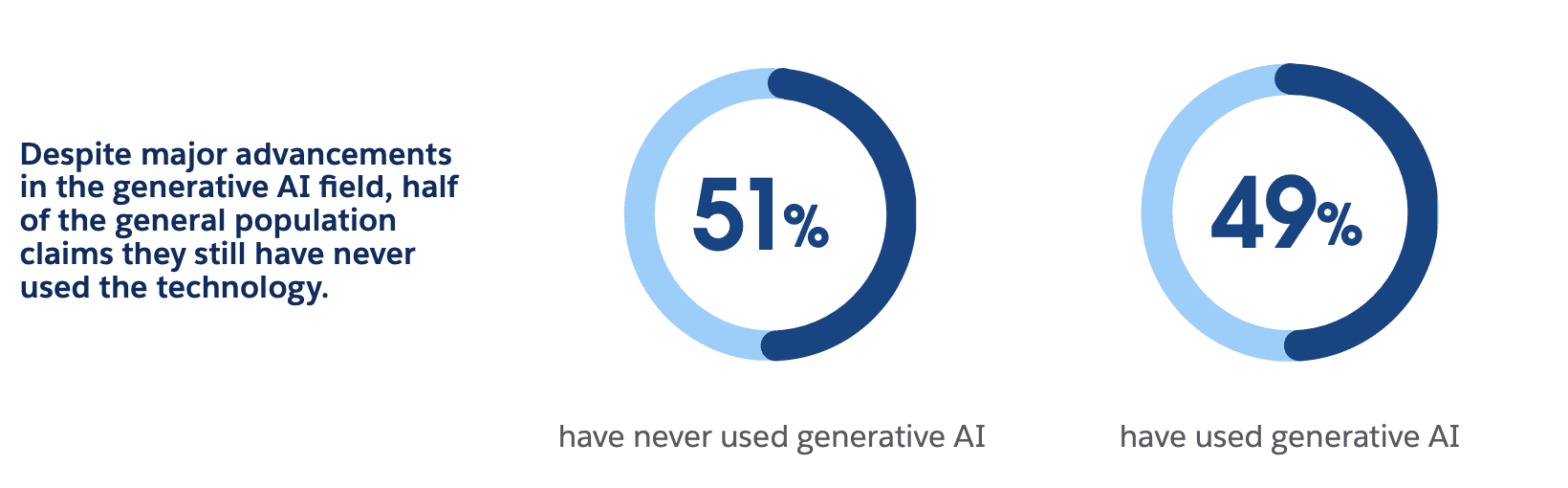
For IT departments, Shadow AI represents a complex dilemma: balancing productivity gains with substantial risks. AI applications frequently require data inputs, some of which could contain sensitive information. Without governance, this data could be inadvertently shared or stored externally, exposing organizations to privacy violations, data leakage, and cybersecurity breaches. The generative AI boom means these tools are no longer a rarity—they’re quickly becoming a daily feature in workplaces. As employees increase their reliance on unsanctioned AI, organizations find themselves unprepared for the evolving risks.
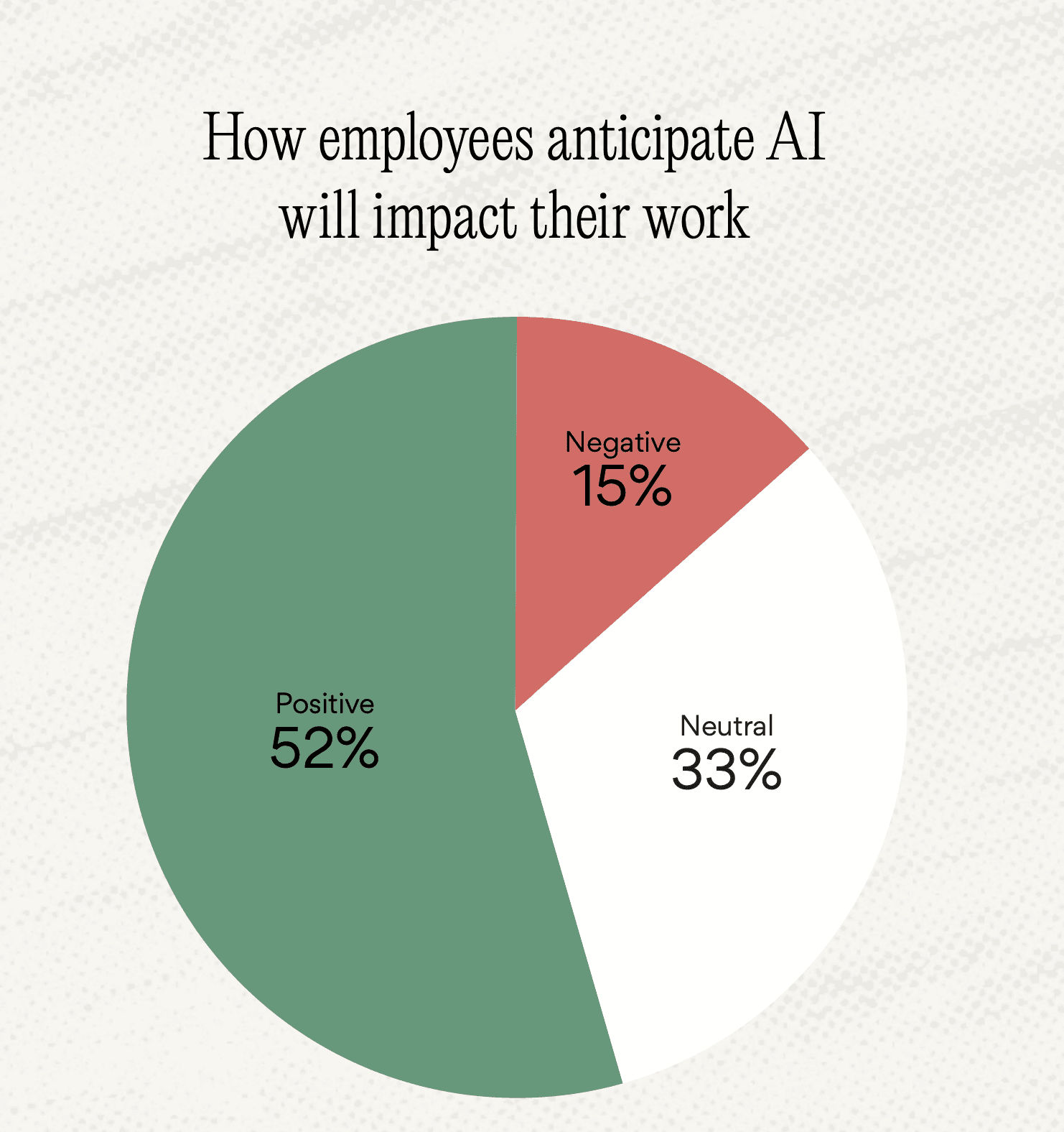
The Urgent Need for AI Governance
So, how can organizations address this hidden yet accelerating threat? Just as Shadow IT forced enterprises to rethink their approach to software governance, Shadow AI now calls for a robust and proactive AI governance framework. Enterprises can control Shadow AI's spread by establishing clear policies, raising awareness, and equipping IT teams to detect unauthorized AI. Tackling Shadow AI isn’t just about security; it’s about future-proofing organizations against new threats in a world where AI will increasingly define competitive advantage.
In this article, we’ll uncover the primary risks of Shadow AI and outline strategic steps IT leaders can take to gain visibility and build a safer AI ecosystem within their organizations. As generative AI technology continues to evolve, enterprises need to decide today how they’ll shape the future of their digital landscape—and secure it against unseen threats.
Key Risks of Shadow AI in Enterprise Environments
The rise of Shadow AI in organizations might seem innocuous, even beneficial, at first glance. After all, employees are leveraging innovative tools to boost productivity. However, without oversight, these tools introduce a spectrum of risks that can impact data security, regulatory compliance, and overall cybersecurity. Let’s delve into the primary risks associated with Shadow AI and why they pose significant challenges for enterprise environments.
1. Data Leakage and Privacy Violations
AI tools often require input data to generate relevant responses. Employees using generative AI tools, like ChatGPT or Midjourney, might inadvertently expose sensitive information by inputting proprietary data, client details, or internal communications. While many employees see these tools as harmless aids, they may be unaware that some AI applications store input data on external servers.
For instance, a notable incident involved Samsung employees who accidentally leaked confidential company information, including internal meeting notes and source code, by inputting it into ChatGPT. Once exposed, this data is vulnerable to misuse or leaks outside the company’s control. Such risks intensify in regulated industries like finance or healthcare, where strict rules govern the handling and storage of data. With the majority of organizations having limited visibility over AI usage, unmonitored AI tools are a growing privacy liability.
2. Compliance and Regulatory Risks
Data misuse through unapproved AI tools could lead to substantial fines and legal ramifications in industries governed by privacy regulations like GDPR in Europe or CCPA in California. Shadow AI compounds compliance challenges because many generative AI tools operate outside the strict data protection protocols required by these regulations.
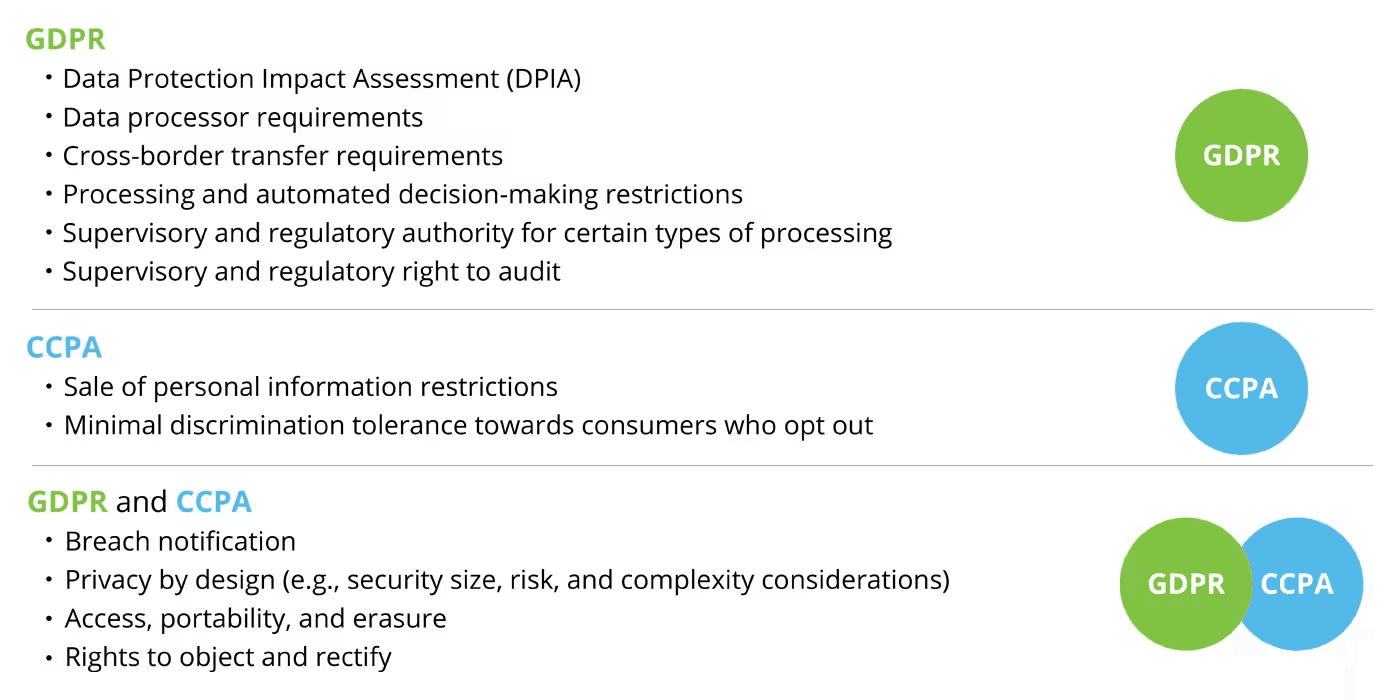
As AI applications generate and process data independently, the chain of data responsibility becomes murky, making it difficult to maintain compliance with privacy and data-handling laws. For example, customer data may be stored, shared, or even embedded within AI algorithms without proper anonymization. This can lead to inadvertent violations of data residency requirements, posing financial and reputational damage to the company.
3. Cybersecurity Threats and Increased Vulnerabilities
While AI applications offer productivity gains, they also expose enterprises to cyber threats. Shadow AI often includes third-party tools that are accessible via the internet, bypassing corporate security protocols. Unlike vetted, secure internal applications, these tools may lack proper encryption, authentication, or data security features, making them potential gateways for cyberattacks.
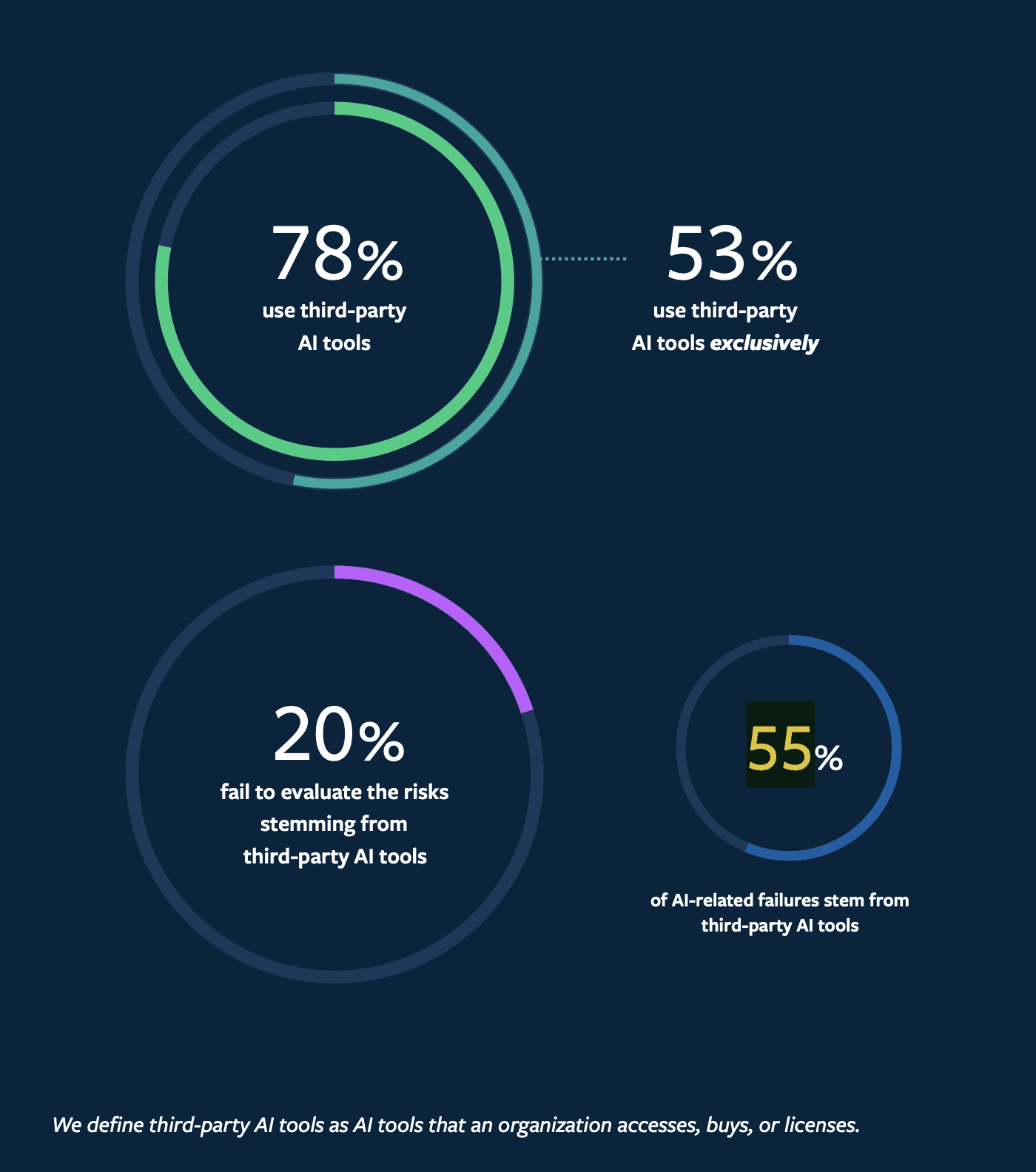
According to recent research, 55% of AI-related failures stem from third-party AI tools, which frequently operate without the enterprise-grade security that internal tools undergo. The open nature of these tools makes them susceptible to unauthorized access, malware insertion, or phishing schemes that exploit employee trust in AI platforms. Cybercriminals may target unmonitored applications, using them as entry points into enterprise networks, creating a significant security loophole that IT teams might not detect until after an attack.
4. Unregulated AI Use Leading to Bias and Unreliable Outputs
Generative AI, when used without proper governance, can lead to unintended biases or inconsistent results that could impact decision-making processes. For instance, if an employee uses an AI tool to help generate hiring recommendations, the lack of control over how the tool was trained or whether it was adequately evaluated for bias can lead to discriminatory or inaccurate results. This poses ethical risks, especially in customer-facing roles or human resources, where biased outputs can lead to unfair treatment or legal repercussions.
The unpredictable nature of generative AI outputs can create liability issues for enterprises. Unlike traditional software that follows defined logic, generative AI models are often trained on vast, uncontrolled datasets, which can introduce implicit biases or errors. Without monitoring, these tools could reinforce biases in hiring, promotions, or customer interactions, harming company reputation and trust.
5. Intellectual Property and Data Ownership Risks
Generative AI tools are designed to produce content—text, images, code—based on user inputs, but the boundaries of intellectual property (IP) rights in these cases can be blurry. Employees may unknowingly use AI tools to produce or transform proprietary information, inadvertently transferring IP ownership to third-party AI providers. This can be particularly problematic in industries relying on IP, such as technology, entertainment, or R&D-driven sectors.
Additionally, some AI tools retain user-generated content to improve their models, a process that often remains hidden from end-users. Without clear data ownership and control policies, companies risk losing control over their IP. Legal battles over ownership and originality could follow, making IP protection a critical, though often overlooked, element of Shadow AI governance.
Visibility and Control Strategies for Shadow AI
To manage Shadow AI effectively, enterprises need a strategy that both uncovers hidden AI tools and provides the oversight needed to protect data and resources. The rapid adoption of generative AI tools demands that IT leaders establish clear protocols for visibility, control, and governance. Here are key strategies to help organizations gain a stronghold on Shadow AI.
1. Implement AI Discovery Tools to Track Unapproved AI Usage
Just as shadow IT discovery tools reveal unsanctioned software, AI-specific discovery tools can detect unauthorized AI applications in an organization’s network. By monitoring software activity, AI discovery tools identify traffic patterns or website interactions associated with AI tools, such as frequently using language models or data-generating applications.
Once detected, these tools can provide a view into which AI platforms are most commonly used and whether sensitive data might be involved. Similar to the oversight applied in traditional IT, this AI-specific tracking gives IT departments the insight they need to assess which tools are safe, which need approval, and which should be restricted.
2. Establish a Cross-Functional AI Governance Committee
Shadow AI crosses multiple organizational boundaries, making it essential to take a cross-functional approach to governance. An AI governance committee can consist of representatives from IT, cybersecurity, compliance, legal, and operations departments. This committee serves as a centralized body to oversee AI policies, assess emerging AI tools, and manage compliance risks.
The committee’s role extends to understanding and evaluating which generative AI tools align with enterprise security and compliance standards. By working collaboratively, the AI governance committee can establish a “safe list” of approved tools while keeping an open line of communication with employees about the proper usage of AI. Regular updates and cross-departmental meetings will help ensure that policies are not only implemented but are adaptive to the fast-paced nature of AI advancements.
3. Set Clear and Accessible Policies on AI Usage
Establishing robust AI usage policies is critical to prevent unauthorized applications from being introduced into the enterprise environment. Effective policies should specify:
Permitted and prohibited AI tools: Provide clear examples of approved tools and specify which are strictly off-limits.
Guidelines on data sensitivity: Define which types of data (such as customer information or financial records) should never be input into AI tools without IT approval.
Safe usage scenarios: List tasks and use cases where employees can leverage AI, such as drafting emails or brainstorming ideas, while prohibiting tasks like handling proprietary data or interacting with client information.
One of the biggest challenges lies in communicating these policies effectively. According to recent research, only 31% of executives believe their AI policies are well-communicated, while just 18% of employees feel they understand the AI usage guidelines. Regular training sessions and easily accessible documentation will help bridge this gap, ensuring that employees feel confident in adhering to safe practices.
4. Encourage a Culture of Transparency and Open Reporting
In many cases, employees use shadow AI tools to meet productivity demands or experiment with innovation, not realizing the risks involved. Organizations can build a non-punitive, open-door policy for AI reporting to foster compliance. By encouraging employees to discuss new AI tools they find useful, IT departments can assess these tools early on, determine their safety, and guide employees toward approved alternatives if needed.
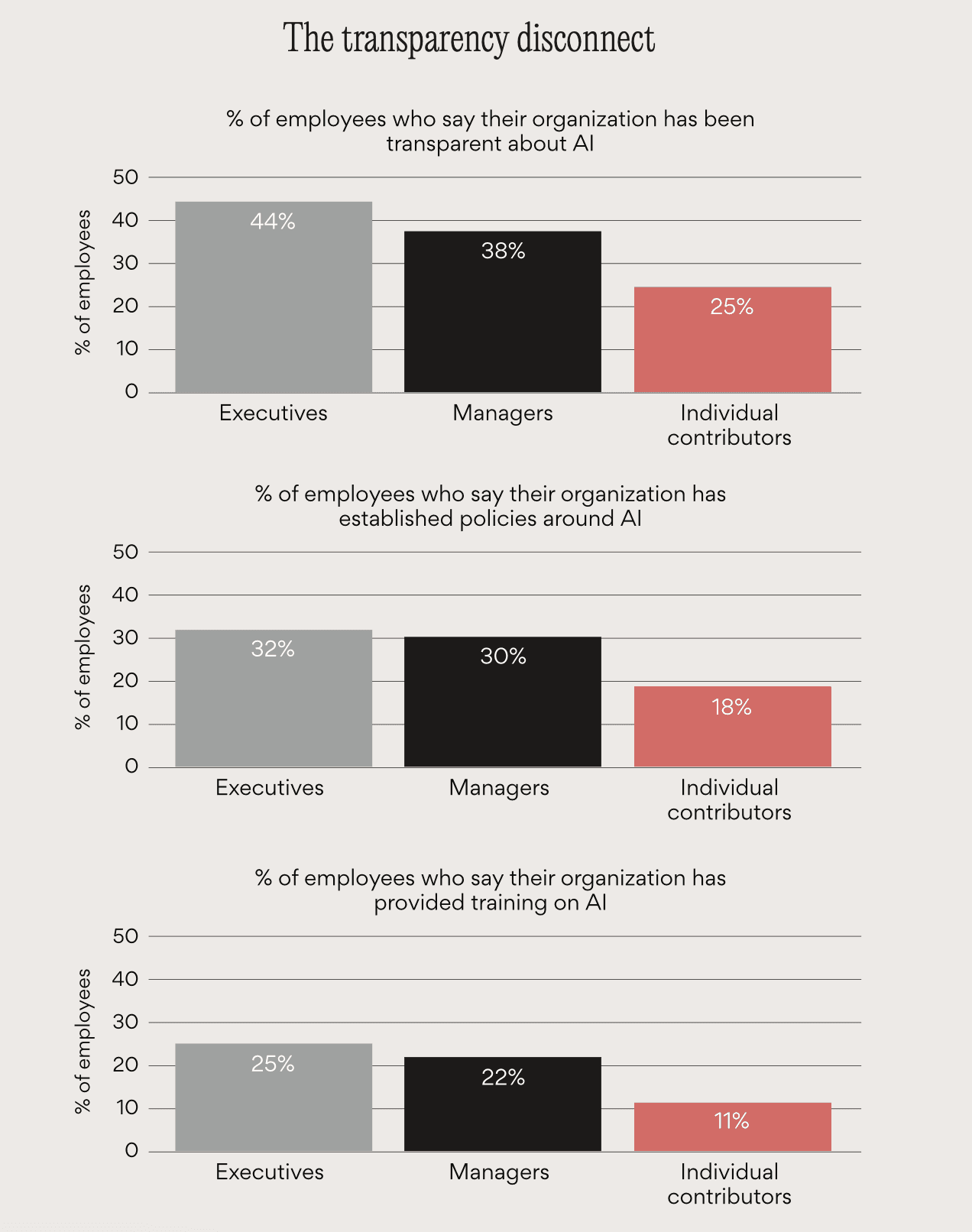
A culture of transparency can also minimize the spread of Shadow AI by making employees aware of the potential repercussions of unauthorized AI use. In addition to regular training, companies can establish “AI champions” within departments who help guide colleagues on compliant AI usage, share updates on approved tools, and provide feedback to IT on evolving needs.
5. Leverage Technology to Restrict High-Risk AI Tools
For enterprises facing frequent Shadow AI challenges, more active control measures can be implemented, such as firewalls and VPNs to restrict access to unauthorized AI sites and tools. By blocking access to specific high-risk websites or applications known for AI-based functionality, IT can reduce the likelihood of unapproved tools penetrating the network. Many organizations are also implementing data loss prevention (DLP) solutions that flag when employees attempt to input sensitive data into unauthorized applications, providing another layer of security.
This proactive approach offers an automated safeguard against unintentional data leakage. With DLP and firewall restrictions in place, IT teams can monitor any attempt to circumvent protocols and continuously improve their control over shadow AI.
6. Regularly Review and Update the AI Governance Strategy
Shadow AI isn’t a static threat; as AI technology advances, new tools will emerge that challenge existing policies. Regular audits and policy updates should be part of every AI governance strategy. The AI governance committee should meet quarterly to assess the current landscape, evaluate which tools employees are adopting, and adjust policies to reflect evolving risks.
These audits allow organizations to remain proactive, refining their approach to AI as new tools and challenges arise. By establishing continuous improvement practices, IT departments can stay ahead of the curve, balancing innovation and risk mitigation to ensure AI tools support rather than threaten organizational goals.
Strengthening AI Governance to Mitigate Risks
Shadow AI isn’t just a new operational challenge; it’s a strategic risk that demands robust governance. To lead their organizations safely through this AI revolution, cybersecurity, IT, cloud, and tech leaders must build a governance framework that aligns AI initiatives with security, compliance, and business objectives. Here’s how to strengthen AI governance to address Shadow AI risks effectively.
1. Define and Enforce a Clear AI Usage Policy Across the Organization
One of the foundational steps in governing AI is creating a formal AI usage policy that addresses who can use AI, under what circumstances, and with which tools. For enterprise leaders, ensuring that this policy isn’t just a written document but an enforceable standard is essential. Define critical parameters, including:
Approved AI tools and use cases: Provide a curated list of pre-vetted AI tools that meet security and compliance standards, detailing which tasks employees can perform with these applications.
Data usage and access control: Specify the types of data that can and cannot be processed with AI tools. Sensitive information, such as customer records or IP, should be strictly off-limits for unapproved tools.
Risk-tiered guidelines: For each tool, categorize risk levels (low, moderate, high) and outline the security checks or permissions needed based on the tool’s risk tier.
For leaders in highly regulated industries, reinforcing these policies with clear, role-specific guidelines can ensure consistency and clarity. Research shows that only 31% of executives report their AI usage policies are communicated across teams, and only 18% of employees feel they understand AI guidelines. As a leader, continuous communication of these policies and targeted follow-ups with departments can reduce ambiguity and increase policy adherence across the organization.
2. Appoint an AI Governance Committee to Oversee Policy Implementation
Managing Shadow AI risks isn’t a one-department job—it requires collaboration across cybersecurity, IT, legal, and compliance. To bring these perspectives together, appoint an AI governance committee tasked with monitoring AI usage, enforcing policies, and conducting risk assessments.
This committee should take a proactive role, regularly evaluating new AI applications to decide which ones align with organizational standards. By setting up this body as a standing committee with executive sponsorship, leaders ensure consistent oversight and centralized governance for all AI-related initiatives. Some of the committee’s core responsibilities can include:
Regular AI audits: Conduct audits of departmental AI usage to ensure compliance and to detect any shadow AI tools in use.
Risk assessments for new AI tools: Evaluate new AI tools for security vulnerabilities, data handling practices, and regulatory compliance before granting them approval.
Cross-departmental training and awareness: Partner with HR and department heads to disseminate safe AI usage practices and update employees on the approved AI tool list.
This committee enforces AI governance and serves as an advisory body, guiding leadership on emerging AI trends and potential policy adaptations.
3. Integrate AI Governance into Cybersecurity and Cloud Strategies
For IT and cloud leaders, it’s critical to weave AI governance into your broader cybersecurity and cloud strategies. AI applications, particularly those accessed through the cloud, present unique challenges due to their rapid adoption and potential data access outside of traditional network perimeters. To mitigate risks, consider:
Securing AI applications on the cloud: Implement identity and access management (IAM) practices specific to AI tools to control which employees can access sensitive applications. Ensure that data processed in the cloud by these AI tools adheres to encryption and data governance standards.
Aligning with cybersecurity protocols: Use established cybersecurity frameworks, such as NIST’s AI Risk Management Framework or ISO/IEC standards, to integrate AI risk mitigation into existing cybersecurity practices. This ensures that AI tools comply with organizational security standards.
Setting up real-time monitoring for high-risk AI activities: In collaboration with cybersecurity teams, establish monitoring protocols that flag unauthorized access to high-risk AI applications or sensitive data uploads.
This integration makes AI governance a seamless part of your existing security infrastructure, reducing exposure and enforcing policies without interrupting productivity.
4. Implement Robust Training Programs for Responsible AI Use
A key component of AI governance is ongoing education, empowering employees to understand both the opportunities and risks of AI. For leadership, investing in comprehensive training programs that teach responsible AI use is essential to minimizing Shadow AI incidents. Training should cover:
Data privacy and security basics: Ensure employees understand data sensitivity and the importance of protecting customer and proprietary information when using AI.
Role-based AI guidelines: To make guidelines more relevant and actionable, create specific training modules that address the AI needs of different departments (e.g., HR, marketing, engineering).
Scenario-based training: Use real-world examples and role-playing scenarios to illustrate potential risks. For instance, show how a seemingly harmless request to ChatGPT could result in a data leak if sensitive information is involved.
By providing targeted, hands-on training, leaders ensure that employees are aware of AI policies and feel equipped to use AI responsibly.
5. Establish Continuous Improvement Protocols for AI Policy Review
AI technology evolves rapidly, and governance frameworks must keep pace. Leaders should set up a regular review process for AI policies, ideally through quarterly or biannual assessments by the AI governance committee. Key components of continuous improvement include:
Quarterly risk assessments and audits: Evaluate whether existing AI tools are functioning within policy parameters and identify any new shadow AI tools in use.
Feedback channels for employees: Encourage employees to report any new AI tools they encounter or provide suggestions for improvements to AI policies.
Policy updates based on regulatory changes: As AI regulations develop globally, from the EU’s AI Act to the White House’s AI Bill of Rights, policies must be adjusted to remain compliant with evolving standards.
Building a structured, continuous review process into your governance framework allows organizations to stay agile, adapt to emerging AI challenges, and reinforce security and compliance.
Conclusion: Turning Shadow AI into a Strategic Asset
Shadow AI may initially seem like an unavoidable risk, but it can be transformed into a powerful asset with a proactive and strategic approach. For IT, cybersecurity, and tech leaders, Shadow AI isn’t simply a problem to solve—it’s an opportunity to shape a secure, innovative future where AI serves both organizational goals and data integrity.
The key lies in building an AI governance framework that minimizes risks and supports responsible AI adoption across the organization. Leaders can shift the AI conversation from reactive control to active enablement with effective policies, cross-departmental oversight, and ongoing training. Employees equipped with the tools and the knowledge to use AI safely will feel empowered to explore AI’s potential without compromising on security.
By integrating AI governance into cybersecurity and cloud strategies, enterprises can position AI as a strategic advantage, gaining visibility and control over the tools employees find useful. In this way, the organization’s approach to Shadow AI becomes a competitive differentiator, balancing innovation with security in a way that inspires employee confidence and organizational trust.
As AI technologies evolve, leaders who address Shadow AI head-on will be prepared to meet the next wave of AI-driven change. Rather than waiting for Shadow AI to turn into a full-fledged risk, seize this moment to foster a resilient AI environment. With thoughtful governance, Shadow AI can move from a hidden liability to a catalyst for responsible innovation, giving your enterprise the agility and security to stay ahead in the AI era.